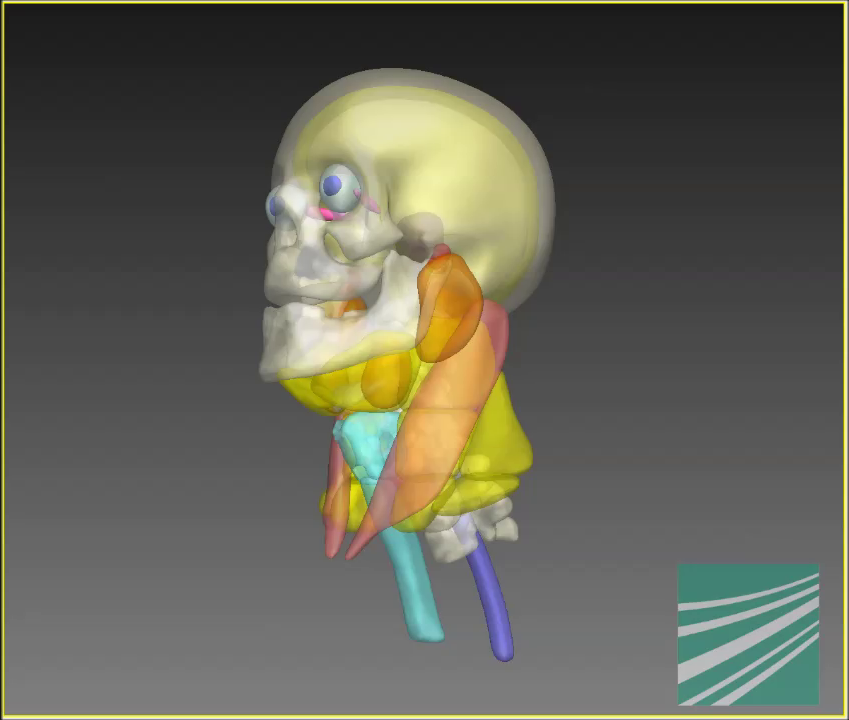
Medical imaging data contains anatomical information that, given the volume of data and especially with radiological 3D imaging data, can no longer reasonably be marked/contoured by hand. The challenge for automatic processes is that not all anatomical regions stand out from their surroundings through strong differences in contrast. Nevertheless, radiologists are able to assign such regions in the imaging data. For automatic algorithms, this requires representing the anatomical knowledge of a clinical expert in a form that is comprehensible to the computer.
To do this, we have developed a generic framework that allows different modalities of medical imaging data (e.g., CT, MRI, photograph) as well as various areas of the body (e.g., head/throat, lower extremities, teeth) to be segmented based on models. The coupled shape model (CoSMo) developed for this contains coupled anatomical structures and represents them in terms of their form and size as well as of their relative position to each other. This makes it possible to reproduce a medical expert’s excellent anatomical knowledge: Just as an expert uses anatomical landmarks for orientation when analyzing and interpreting imaging data, CoSMo can localize the region in which a certain structure is expected in the imaging data by using its learned relative position to surrounding structures.
In the developed framework, the representation of the model, its training based on available reference segmentations and the adaptation strategy for automatic segmentation are controlled using textual configuration files. These XML-based, hierarchically structured text files are easy to read - both by a computer, given the large number of XML implementations available, and by the human user who needs to enter the application-specific parameters into the XML files. This separation of basic algorithms and application-specific parameters allows CoSMo to be applied to new problems - image modalities or body areas - with little effort.