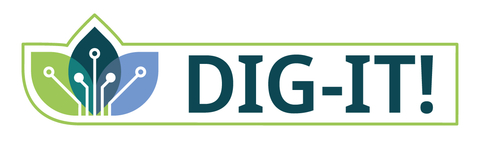
Ecological sciences frequently generate large amounts of imagery to address issues such as ecosystem stability in the face of climate and land-use changes, species conservation, and environmental monitoring. For example, camera traps are used to observe animal behavior, minirhizotrons to analyze plant root growth, and microscope images of thin wood sections to read weather conditions in recent years. Currently, this image data must be analyzed manually by experts in an extremely time-consuming process.
The DIG-IT! project addresses these ecological issues from a digitization perspective. The goal is to make the advances in machine vision over the last decade, in particular deep learning, available to the field of ecology. The applications mentioned above are used for purposes such as identifying and classifying bats by species and measuring wood cells and plant roots. The entire process must be as automated as possible and require little input from human experts. To achieve this, developers are focusing on universally applicable methods using self-learning algorithms (deep convolutional neural networks), which will be provided to the scientific community as a user-friendly toolkit.
The DIG-It! collaborative project combines developer expertise in automated analysis of image data (Fraunhofer Institute for Computer Graphics Research Rostock and Biomathematics, University of Greifswald) with applications in urgent ecological issues (Botany/Landscape Ecology/Zoology, University of Greifswald.